Introduction
The integration of Artificial Intelligence (AI) in agriculture is transforming traditional farming practices into precise, data-driven processes. From optimizing crop yield to monitoring plant health, AI technologies enable real-time solutions for complex agricultural problems. This article delves into 10 advanced use cases where AI addresses specific challenges in agriculture, providing technical insights and references to support recent research findings.
AI in Agriculture: An Overview
What is AI in Agriculture?
AI in agriculture refers to the use of machine learning, computer vision, deep learning, and predictive analytics to address challenges related to food production, sustainability, and farm management. AI models process vast amounts of agricultural data to provide insights that guide decisions, predict outcomes, and automate tasks.
AI Use Cases in Agriculture
1. Crop Disease Detection and Classification Using Computer Vision
Problem: Crop diseases can devastate yields, but early detection remains challenging due to scale and complexity. Identifying disease symptoms visually is labor-intensive and prone to inaccuracies.
Solution: Using computer vision and convolutional neural networks (CNNs), AI systems can classify diseases based on images captured by drones or IoT devices. CNNs are trained on large datasets of diseased crop images, learning to differentiate specific leaf spots, blights, and rots. Techniques like Transfer Learning can enhance accuracy, allowing CNNs to be repurposed across multiple crops. For instance, studies indicate that CNN-based models can achieve over 90% accuracy in real-time detection of diseases in crops like tomatoes and wheat source.
2. Precision Weed Control with Machine Learning and Robotics
Problem: Traditional herbicide spraying treats entire fields, leading to excessive chemical use, environmental harm, and increased costs.
Solution: AI-powered robotics, coupled with machine learning algorithms, enable precision weed control by distinguishing between crops and weeds. Semantic segmentation algorithms segment each plant pixel in the image, helping identify weeds with accuracy. AI-equipped drones or autonomous robots can then spray herbicides only where necessary. Studies show a reduction of up to 90% in herbicide use, significantly lowering costs and environmental impact source.
3. Yield Prediction Using Deep Learning and Climate Data
Problem: Predicting crop yield accurately is essential for food supply management but is affected by climate variability and other factors.
Solution: Deep learning models, especially recurrent neural networks (RNNs) and long short-term memory (LSTM) networks, analyze past yield data, soil health metrics, and climate forecasts to predict future yield. By training these models on historical yield and environmental data, they can learn complex patterns and provide precise yield forecasts. Research demonstrates that LSTM-based models improve yield prediction accuracy by up to 25% compared to traditional models source.
4. Soil Health Monitoring and Nutrient Prediction Using IoT Sensors and AI Models
Problem: Soil health is critical for crop productivity, but assessing nutrients and moisture levels across large fields manually is inefficient.
Solution: By deploying IoT sensors that measure soil moisture, pH, and nutrient levels, and coupling them with AI algorithms, farmers can monitor soil health in real-time. Using regression models and anomaly detection algorithms, AI can analyze soil data to predict nutrient deficiencies, optimizing fertilizer use. Studies show a 15-20% improvement in yield when soil health is monitored with AI-driven predictions source.
5. Pest Detection and Control with Predictive Analytics and Image Recognition
Problem: Pests can quickly destroy crops, requiring rapid identification and control.
Solution: AI models, particularly image recognition software, use pest imagery to detect infestations. Combined with predictive analytics, models analyze patterns of pest outbreaks and alert farmers to potential threats. Bayesian networks and support vector machines (SVMs) are particularly effective for prediction and classification, reducing crop losses by up to 30% by ensuring timely interventions
source.
Additional Use Cases
6. Irrigation Optimization Using Neural Networks and Weather Forecasting
7. Seed Planting and Crop Spacing Optimization with Reinforcement Learning
8. Quality Sorting and Grading of Produce with Deep Learning Models
9. Crop Rotation Planning Using Genetic Algorithms
10. Supply Chain Optimization with AI-Driven Forecasting Models
Each of these use cases demonstrates how AI transforms agriculture, leading to smarter, more sustainable, and profitable farming practices. AI in agriculture has shown remarkable potential, with studies predicting an annual growth rate of 25% for AI in this sector, underscoring its critical role in addressing food security challenges globally source.
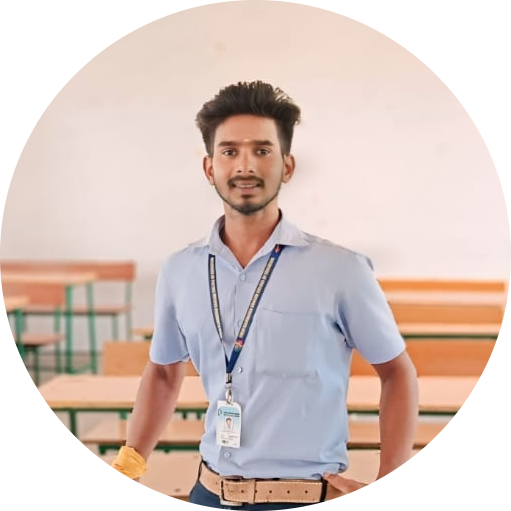
ABOUT AUTHOR
Venkateshkumar S
Full-stack Developer
“Started his professional career from an AI Startup, Venkatesh has vast experience in Artificial Intelligence and Full Stack Development. He loves to explore the innovation ecosystem and present technological advancements in simple words to his readers. Venkatesh is based in Madurai.”